Using this method, MIT researchers aim to unify data from diverse domains into a common language that AI models can understand.
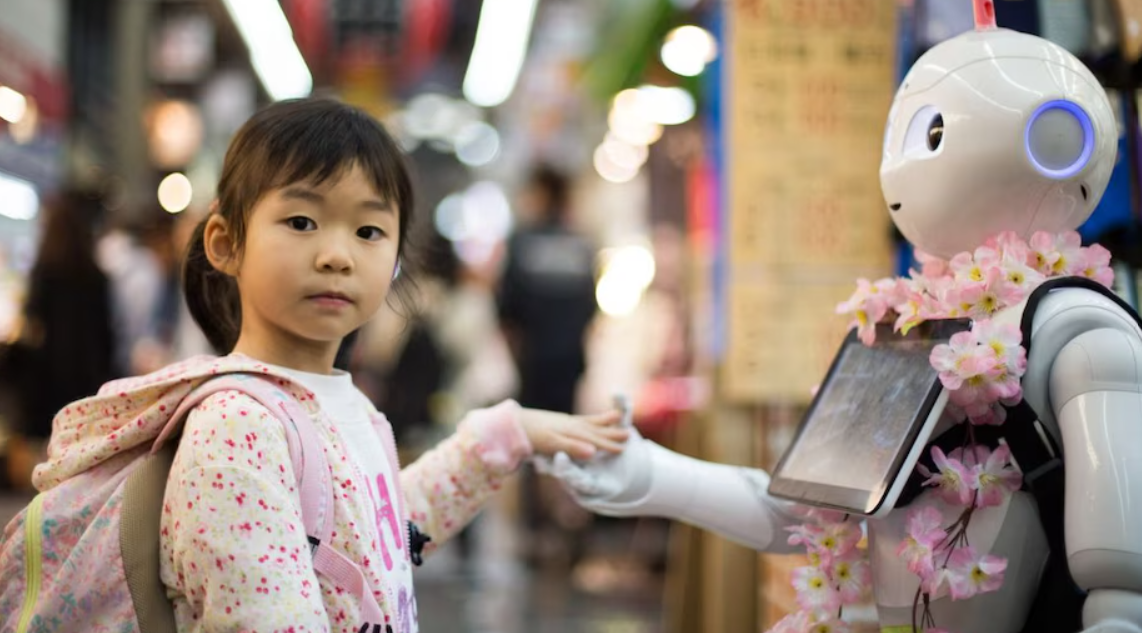
To unify data from different domains, MIT used Heterogeneous Pretrained Transformers (HPT) architecture
Last week, the Massachusetts Institute of Technology (MIT) introduced a novel approach to training robots that leverages generative artificial intelligence (AI) models. This technique integrates data from various domains and modalities into a unified language that large language models (LLMs) can process. According to MIT researchers, this method could enable the development of general-purpose robots capable of performing a wide array of tasks without the need to train each skill individually from the ground up.
MIT Researchers Develop AI-Inspired Technique for Robot Training
In a recent announcement, MIT unveiled a groundbreaking methodology for training robots. Currently, teaching a specific task to a robot is a complex challenge, as it requires extensive simulation and real-world data. This is crucial because if a robot cannot comprehend how to perform a task in a particular environment, it will struggle to adapt.
This means that each new task requires fresh data that covers a wide range of simulations and real-world scenarios. The robot then goes through a training phase, where actions are optimized, and any errors or glitches are corrected. As a result, researchers typically train robots for specific tasks. The adaptable, multi-purpose robots depicted in science fiction movies have not yet become a reality.
Researchers at MIT have developed a new technique that may overcome this challenge. In a paper published on the pre-print platform arXiv (not yet peer-reviewed), the researchers propose that generative AI could help address this issue.
The approach involves unifying data from different domains, such as simulations and real robots. It also integrates various types of inputs, including vision sensors and robotic arm position encoders. These inputs are unified into a common “language” that an AI model can process. The team also developed a new architecture called Heterogeneous Pretrained Transformers (HPT) to handle this unified data.
Interestingly, Lirui Wang, the lead author and a graduate student in electrical engineering and computer science, made an observation. He noted that this technique was inspired by AI models. Specifically, he referenced OpenAI’s GPT-4 as a key influence.
The researchers integrated a transformer-based large language model (LLM), similar to the GPT architecture, into the core of their system. This addition enables the model to process both visual inputs and proprioceptive inputs, which include the senses of movement, force, and position.
According to the MIT researchers, this new method could make training robots faster and more cost-effective than traditional approaches. This efficiency comes from needing significantly less task-specific data to train the robot for various tasks. Additionally, the study showed that this method outperformed training from scratch by over 20 percent in both simulations and real-world tests.